Synergistic use of optical and SAR data for the retrieval of essential climate and agricultural variables
- Project by: Jean Bouchat
- October 29, 2024
Earth observation (EO)-based optical vegetation descriptors—such as the leaf area index (LAI), the fraction of absorbed photosynthetic active radiation (fAPAR), the fraction of green vegetation as seen from nadir (fCover), and the canopy chlorophyll content (CCC)—are essential for monitoring key processes including photosynthesis, respiration, transpiration, rainfall interception, and surface energy balance. These biophysical variables are crucial for evaluating the growth, health, and productivity of crops and, more broadly, vegetation. The importance of LAI and fAPAR has been widely recognized for the continuous monitoring and modeling of the Earth’s surface. As such, they have been designated as essential climate variables (ECVs) by the Global Climate Observing System (GCOS), and their estimation has been the subject of considerable effort by the land remote sensing community.
Most large-scale and cost-effective methods for estimating these biophysical variables currently rely on optical remote sensing data. However, frequent cloud cover, which obscures approximately 70% of the Earth’s surface at any given time, can significantly hinder their reliability. This challenge is particularly pressing in tropical regions, where timely vegetation monitoring is essential for food security and where cloud cover often overlaps with key phases of vegetation growth. In these instances, Synthetic Aperture Radars (SARs) offer a valuable alternative, as their cloud-penetrating capabilities enable the generation of dense time series that can enhance the spatial and temporal coverage of optical data.
In recent years, various methods have been proposed to address gaps in the time series of these optical vegetation descriptors. Notably, fusion techniques have been developed to reproduce or enhance optical imagery using SAR data. These methods often involve predicting missing reflectance values by mapping SAR data to optical images, frequently utilizing deep learning models to capture the complex relationships between the two data sources. However, there have been relatively few efforts to systematically leverage dense SAR time series to directly fill past or current gaps in optical vegetation descriptor time series, though this could prevent the accumulation of modeling errors and reduce production time.
This research project focuses on the development of methods for synergistic SAR-optical biophysical variables regression to fill gaps in their time series as they occur. Based on very promising results already obtained for maize in Belgium, we intend to extend the approach to the other aforementioned biophysical variables, to all major rainfed crops, and in a wide range of cropping systems, with particular attention to cloud-prone regions where optical systems remain blind during critical periods. Ultimately, our aim is to improve the reliability of operational vegetation monitoring systems, such as early warning systems for crop stress, and bring us a decisive step closer to the Digital Twin Earth.
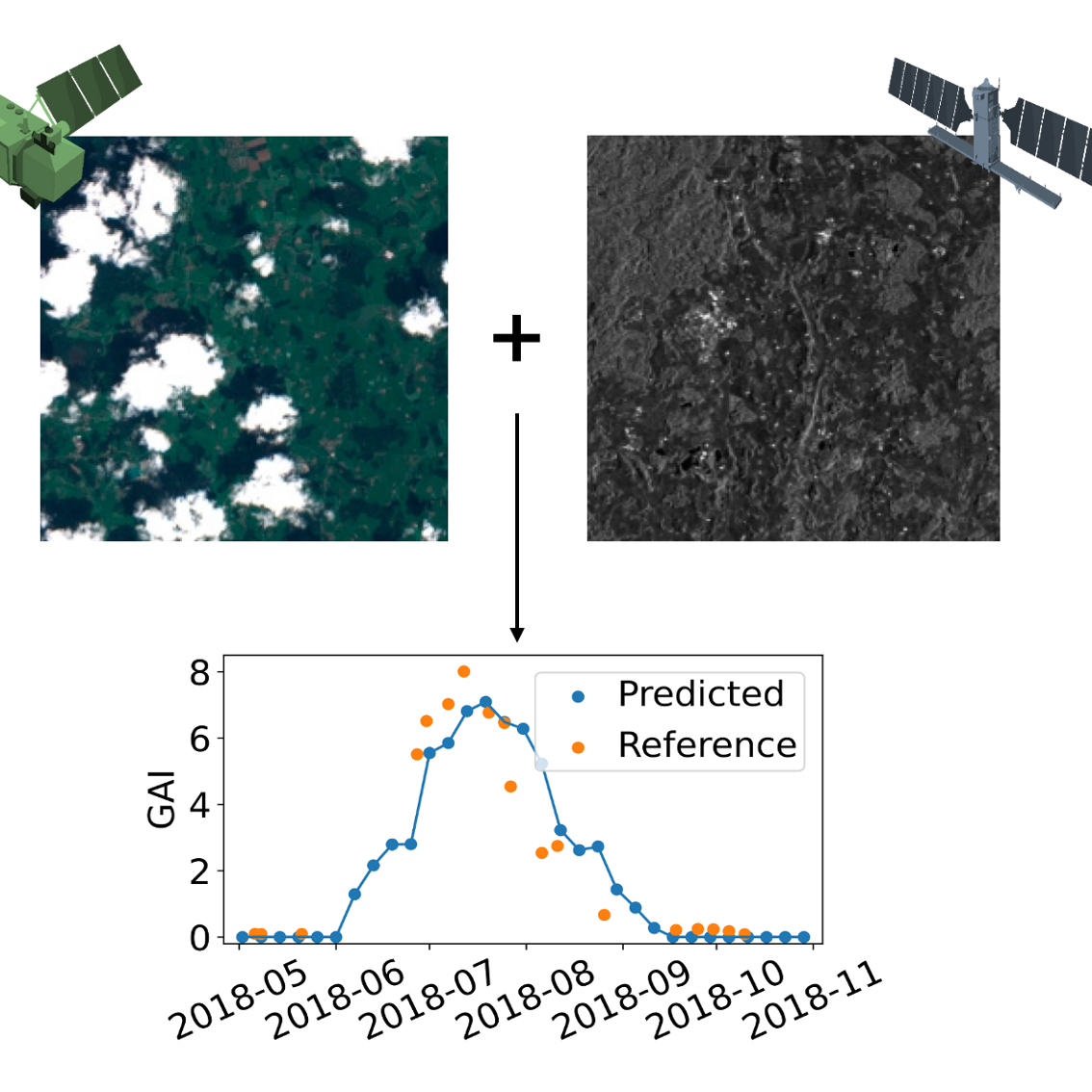
This research is carried out by Jean Bouchat.